In an era where artificial intelligence is revolutionizing creative industries, understanding how to correctly generate an image using AI is no longer a specialist's realm—it’s a necessity for anyone engaged in digital content creation. Image generation using AI can help streamline workflows, enhance creativity, and break down creative barriers if done correctly. With the right approach, AI can transform raw ideas into stunning visual representations seamlessly.
How to Correctly Generate An Image Using AI
To correctly generate an image using AI, it's imperative to start with a clear understanding of the tools and processes involved. AI image generation typically involves leveraging machine learning models like Generative Adversarial Networks (GANs) or diffusion models, which can produce highly detailed and accurate images.
These models require extensive data input and training, making data pre-processing a critical step. By ensuring your dataset is clean, diverse, and accurately labeled, you significantly enhance the quality of your AI-generated images. Key aspects such as choosing the right model and configuring it according to specific requirements are vital for producing the desired output.
Let’s break down the steps on how to correctly generate an image using AI:
- Define Your Objectives: Clearly outline what you aim to achieve with AI-generated images. Whether it's for marketing, architectural design, or digital art, clarity in your objectives will guide your choice of AI tools and datasets.
- Select the Right Model: Depending on your goal, choose between GANs, transformers, or other AI models. For photorealistic images, GANs are often preferred. For style transfer or artistic expressions, other models might be more suitable.
- Data Preparation: Assemble and preprocess a robust dataset. This means cleaning the data, annotating images properly, and ensuring a diverse set of samples to teach the AI model effectively.
- Model Training and Tuning: Configure the parameters and fine-tune the model with the dataset to achieve optimal performance. This may involve iterative testing and adjustment to get the desired image fidelity.
- Evaluate & Refine: Continuously evaluate the output using metrics and human feedback. Adjust the model as necessary to enhance image quality and realism.
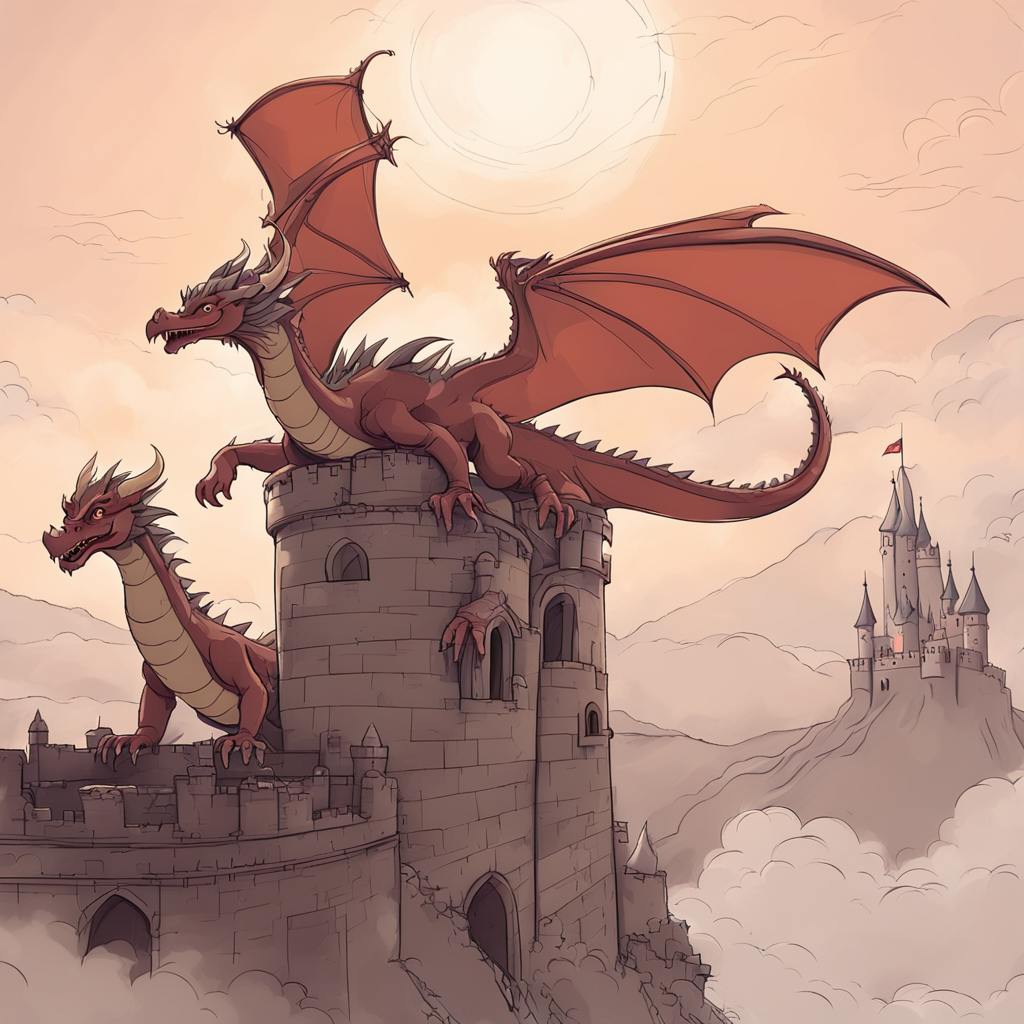
AI made with Mili Fay
The Potential of AI In Image Generation
The transformative potential of AI in image generation is compelling, offering innovative ways to visualize concepts that would be laborious or impossible manually. AI breaks down creative barriers by offering tools that can interpret and materialize abstract concepts into vivid images. By automating mundane tasks, it empowers creatives to focus more on ideation and innovation. For example, digital marketers can swiftly generate compelling visuals tailored for different demographics and cultures by refining model inputs, significantly enhancing engagement and conversion rates.
Furthermore, AI democratizes image creation, making high-quality visuals accessible to individuals and small businesses without the resources typically required for traditional methods. This level of accessibility enhances the diversity of creative voices and fosters innovation across industries.
The Application of AI In Image Generation
The application of AI in image generation is underpinned by rigorous scientific research and widespread industry adoption. Technologies like GANs and transformers have been the subject of extensive study, leading to numerous enhancements that make these models more efficient and effective. Credible AI platforms like DeepArt, RunwayML, and OpenAI’s DALL-E 2 provide powerful tools backed by a strong track record of success in various applications, from entertainment to ecommerce. By leveraging these reputable tools, users can generate high-quality images that meet professional standards.
FAQ: How to Correctly Generate Images Using AI
What is the Correct Method for Generating Images Using AI?
The correct method for generating images using AI involves several key steps, typically performed in sequence using deep learning models such as Generative Adversarial Networks (GANs), Variational Autoencoders (VAEs), or Diffusion Models:
- Data Collection and Preparation: Start with collecting a large and diverse dataset of real images relevant to the desired output. This dataset should be preprocessed to ensure uniform quality and sizing, reducing noise and enhancing features suitable for training.
- Model Selection: Choose a model architecture based on your specific needs. GANs are popular for generating high-quality images, while VAEs are useful for controlled image generation tasks. Diffusion models are gaining popularity for their stability and capability in generating high-fidelity images.
- Training the Model: Train the selected model on the prepared dataset. This step requires a powerful computing environment, as it involves adjusting model parameters through gradient descent to minimize the difference between the generated images and the real images (or another target metric).
- Tuning and Validation: Once trained, fine-tune the model to improve image quality. This involves adjusting hyperparameters, augmenting the data, or introducing novel loss functions to enhance specific image features.
- Image Generation: Use the trained model to generate new images. The generation process can involve tweaking model input (latent space) or using conditional inputs to control specific aspects of the output.
- Evaluation and Iteration: Evaluate the generated images for fidelity and diversity. Methods such as the Inception Score (IS) and Fréchet Inception Distance (FID) are utilized. Further iterations might be needed to enhance outcomes.
What Are Some Common Mistakes to Avoid When Generating Images with AI?
- Insufficient Data Quality or Quantity: Using a small or low-quality dataset can hinder the model's ability to generate realistic images.
- Inadequate Model Training: Skipping thorough training due to computational cost or time can result in poor-quality images. Overfitting or underfitting are also common issues that deteriorate image quality.
- Ignoring Preprocessing Steps: Neglecting to properly preprocess images can introduce artifacts or inconsistencies in the generated images.
- Overlooking Hyperparameter Tuning: Failing to adjust learning rates, batch sizes, or other hyperparameters can negatively affect the model’s performance.
- Lack of Evaluation: Not using objective metrics to evaluate the quality and diversity of generated images might lead to subjective judgments about improvements in the generation process.
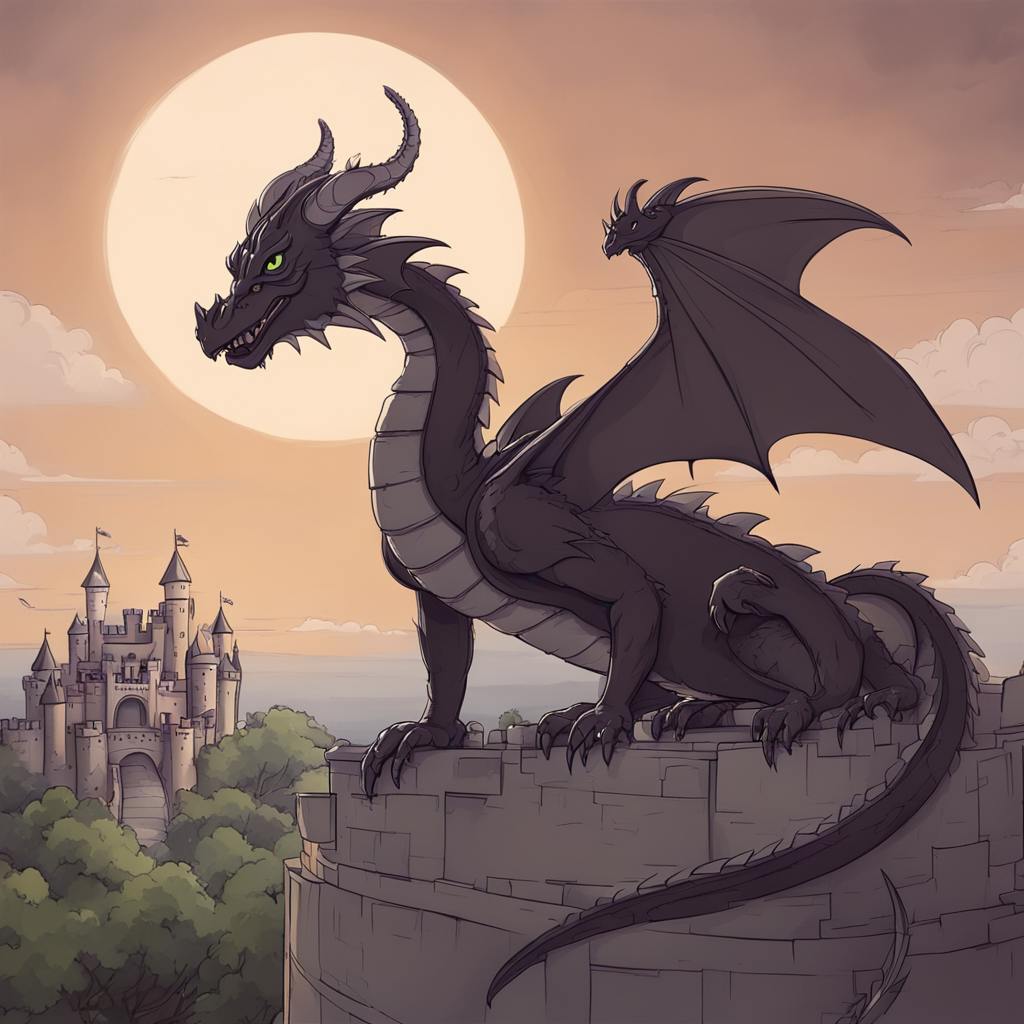
AI made with Mili Fay
Why is a Specific Method Recommended for Image Generation Using AI?
Different AI models and techniques are recommended based on the specific goals and constraints of the image generation task. Here’s why:
- GANs: Recommended for high-quality image synthesis due to their adversarial learning framework, which pits two networks against each other, resulting in highly realistic images.
- VAEs: Useful for applications needing structured latent spaces and generating images with specific variations and attributes. They often are preferred when interpretability is required.
- Diffusion Models: Their progressive denoising framework provides robust stability and high-quality image outputs, making them an increasingly popular choice in balancing fidelity and diversity.
Each method has been optimized for varying trade-offs between quality, control, and computational resources, making them suitable for different applications of image generation in both academic research and industrial settings.
Conclusion
Generating images using AI—when done correctly—offers unprecedented opportunities to innovate and streamline creative processes. By first understanding the fundamental methods and selecting the appropriate tools, refining data inputs, and iterating results, it is possible to harness AI's transformative power effectively.
This methodology not only enhances the quality of generated images but also expands creative possibilities. By following these steps, you can confidently break down creative barriers, harnessing AI to transform your vision into vibrant reality. Understanding 'how to correctly generate images using AI' will unlock these potentials and keep you at the forefront of technological creativity.